
A Bayesian Appproach To Locally Varying Regularization In Optical Flow Velocimetry
Gauresh Raj Jassal, Erkki Somersalo, Daniela Calvetti, Bryan Eric Schmidt
Case Western Reserve University, Cleveland, United States
DOI:
A recent method for determining accurate, dense velocity fields from a pair of particle images is to solve the optical flow problem, but the ill-posed inverse problem of optical flow velocimetry (OFV) generally entails minimizing a weighted sum of two terms--fidelity and regularization--and the weights in the sum are parameters that require manual tuning based on the flow properties and particle images. This tuning of the weighting parameters makes the experimental applicability of the method much more challenging as the calculated velocity field has demonstrated sensitivity to the value of the weights. This work proposes a hierarchical model for the weighting parameters in the framework of maximum a posteriori (MAP)-based Bayesian optimization approaches. The resulting method is tested on three different synthetic datasets and on experimental particle images. The method is found to be capable of self-adjusting the local weights of the optimization process in real-time while simultaneously determining the velocity field, leading to a more accurate estimate of the velocity field without requiring any dataset-specific manual tuning of parameters.
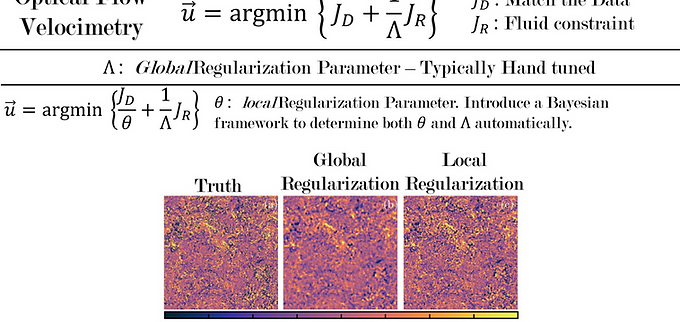